PleioGraph
PleioGraph is a novel statistical method that bridges GWAS and drug discovery. It allows for the detection of clusters of colocalized traits within a vast set of candidate traits. PleioGraph efficiently summarizes these findings in intuitive knowledge-graphs, enhancing insights on prioritization. This approach not only enhances the utility of GWAS results by offering deeper functional insights but also lays the foundation for formulating insightful biological hypotheses.
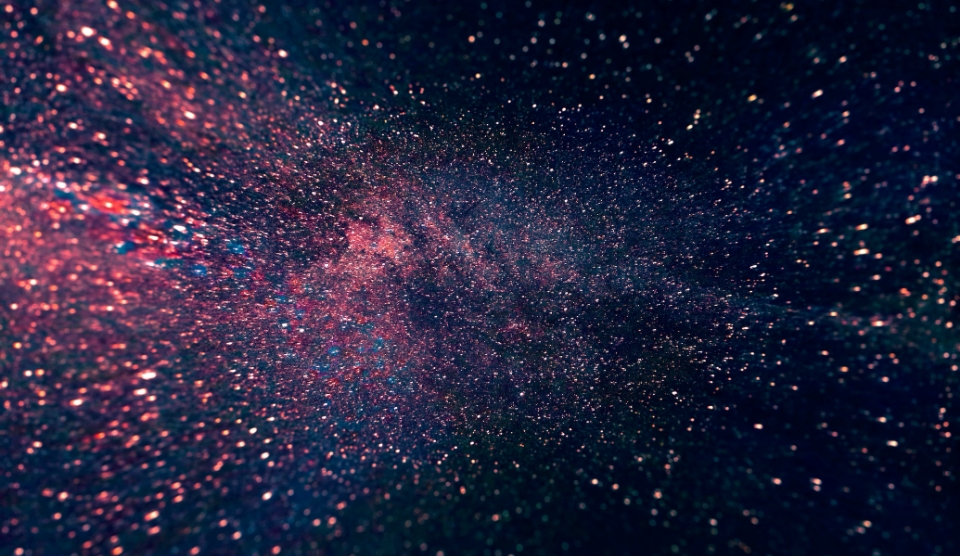
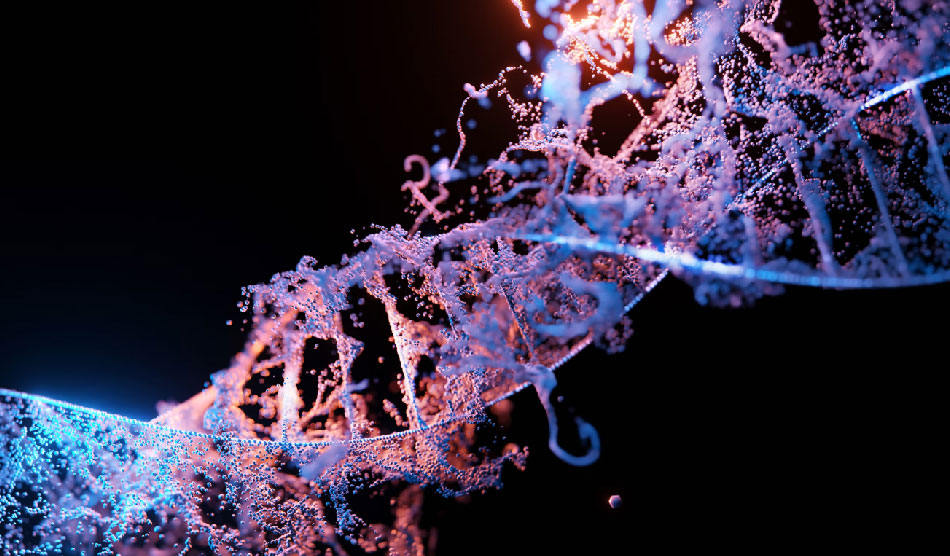
isGWAS
Our cutting-edge in-silico genome-wide association (isGWAS) technique presents a groundbreaking statistical algorithm that transforms the time-consuming nature of traditional GWAS into a matter of minutes. By leveraging isGWAS, researchers can effortlessly examine whole-genome sequencing data from millions of individuals without the necessity of accessing individual-level information. Beyond its computational advantages, isGWAS facilitates biobank design by predicting disease associations for arbitrary sample sizes.
Evolutionary Algorithms
Evolutionary Algorithms (EAs) are a set of generic, population-based algorithms that use heuristic approaches for solving non-trivial challenges such as large-scale imputation. They use natural evolution-inspired mechanisms like selection, recombination, reproduction, and mutation. EAs excel at discovering non-linear relationships between features and optimizing multiple objectives. We make use of our expertise in EAs to help our partners generate insights from their highly heterogeneous datasets.
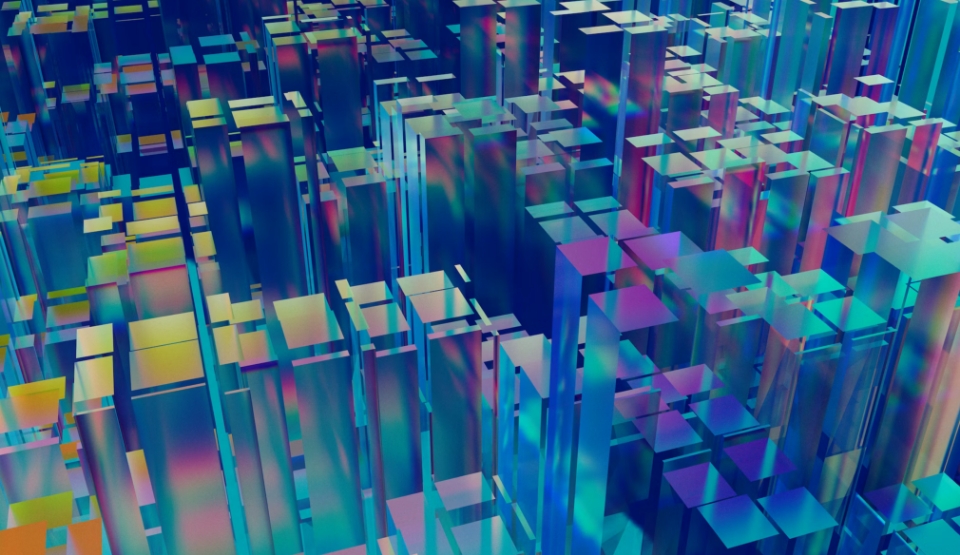
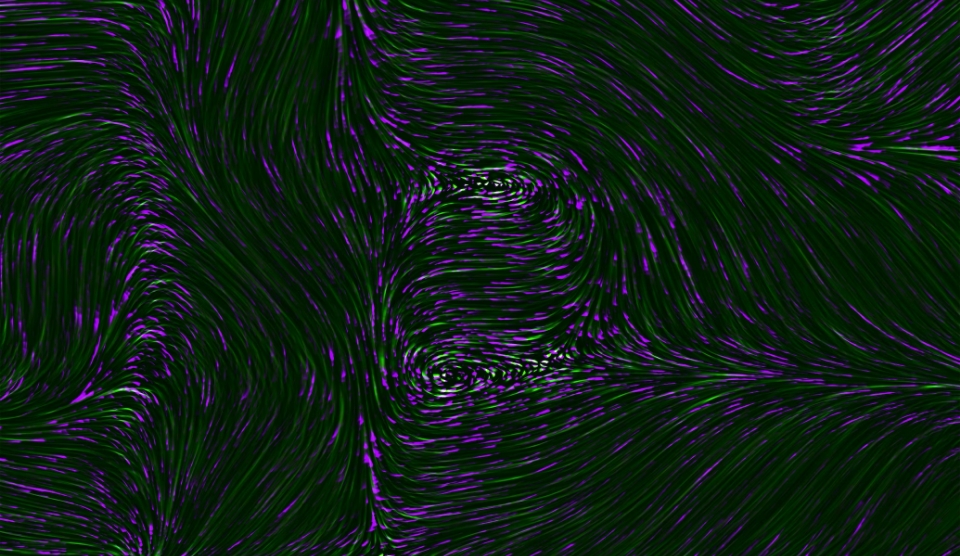
MR-CLUST
With the recent progress in using causal inference in healthcare, which has shown its ability to provide a comprehensive understanding of disease mechanisms, BioXcelerate have created an extension to traditional Mendelian Randomization.
MR-Clust goes beyond traditional MR by accounting for heterogeneity present in interactions between risk factors, biological pathways and provides clusters of genetic variants that uncovers different causal mechanisms while accounting for uncertainty.
Networks and Knowledge Graphs
With the recent progress in using causal inference in healthcare, which has shown its ability to provide a comprehensive understanding of disease mechanisms, BioXcelerate have created an extension to traditional Mendelian Randomization.
Network analysis is fundamental in uncovering insights by integration, interpretation, and visualization of multi-omic biological insights critical for drug discovery, patient diagnostics, and clinical development. Our expertise in clustering and analysis of networks and knowledge graphs provides insights across omic modalities and enables our partners to accelerate insight generation and interpretation.
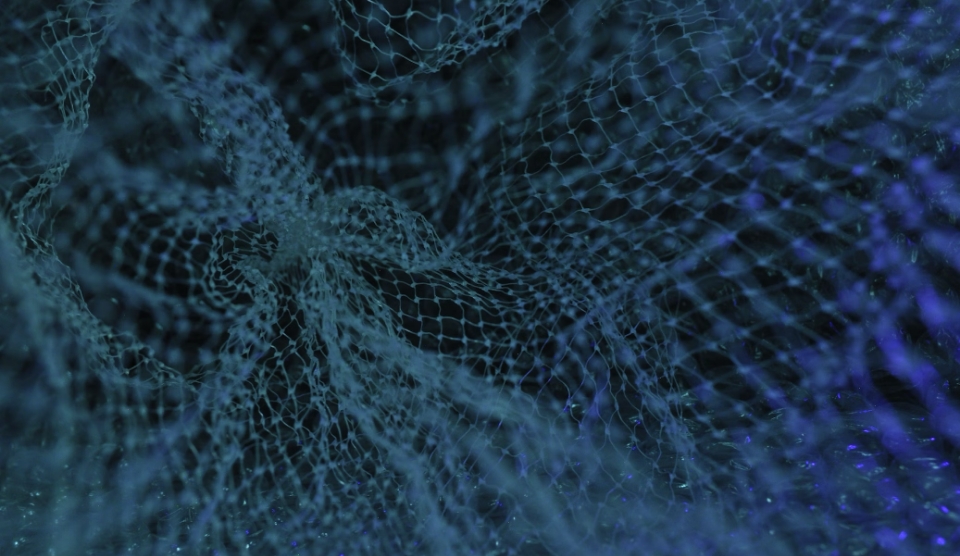